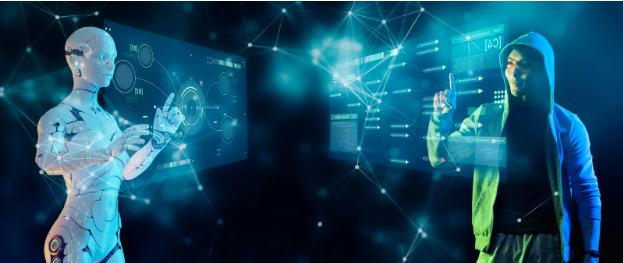
Ransomware attacks have become one of the most significant cyber threats to businesses and organizations of all sizes. According to a report by Cybersecurity Ventures, ransomware is expected to cost businesses more than $20 billion globally by 2021.
These attacks are not only costly but can also result in the loss of sensitive data, which can have serious consequences for businesses.
To tackle this issue, companies are turning to artificial intelligence (AI) and machine learning to help detect and prevent ransomware attacks. In this article, we will explore the role of AI and machine learning in preventing ransomware attacks and the benefits they bring.
What is Ransomware?
Ransomware is a type of malware that encrypts files on a computer system or network and demands payment in exchange for the decryption key. The attackers typically threaten to delete or publish the encrypted data if the ransom is not paid within a specified timeframe.
Ransomware attacks can be delivered via various methods, including email attachments, malicious links, and software vulnerabilities. Once the ransomware is activated, it can quickly spread throughout a network, encrypting files and locking users out of their systems.
How Can AI and Machine Learning Help Prevent Ransomware Attacks?
AI and machine learning can help prevent ransomware attacks by identifying and analyzing patterns in data that may indicate a ransomware attack. They can also help detect and respond to ransomware attacks more quickly and accurately than human operators.
AI and Machine Learning for Anomaly Detection
One of the most effective ways AI and machine learning can help prevent ransomware attacks is through anomaly detection. Anomaly detection is the process of identifying patterns in data that do not conform to the expected behavior or norm. By using AI and machine learning algorithms, companies can identify anomalous behavior that may be indicative of a ransomware attack.
For example, if a user suddenly starts accessing a large number of files that they don't usually access, it could be an indication of a ransomware attack. AI and machine learning algorithms can detect this unusual behavior and alert the security team to investigate further.
AI and Machine Learning for Threat Intelligence
AI and machine learning can also help prevent ransomware attacks by analyzing threat intelligence data. Threat intelligence is the process of gathering information about potential cyber threats, such as known malware variants, attacker tactics, and vulnerabilities. By using AI and machine learning algorithms to analyze this data, companies can identify potential ransomware attacks before they occur.
For example, AI and machine learning algorithms can analyze threat intelligence data to identify known ransomware variants and their characteristics. By doing so, security teams can prepare for potential ransomware attacks and take steps to prevent them.
AI and Machine Learning for Behavioral Analysis
AI and machine learning can also help prevent ransomware attacks by analyzing user behavior. By monitoring user behavior, AI and machine learning algorithms can identify unusual activity that may be indicative of a ransomware attack.
For example, if a user starts accessing files outside of their normal work hours, it could be a sign that they have been compromised by ransomware. AI and machine learning algorithms can analyze this behavior and alert the security team to investigate further.
AI and Machine Learning for Response and Recovery
AI and machine learning can also help organizations respond to and recover from ransomware attacks more quickly and effectively. By analyzing past attacks and their characteristics, AI and machine learning algorithms can help security teams develop more effective response and recovery plans.
For example, AI and machine learning algorithms can analyze data from past ransomware attacks to identify common characteristics and develop effective countermeasures. These countermeasures can include automated responses, such as isolating infected systems, disconnecting them from the network, and alerting the security team.
The Benefits of AI and Machine Learning in Preventing Ransomware Attacks
The benefits of AI and machine learning in preventing ransomware attacks are numerous. Some of the most significant benefits include:
Early Detection and Prevention
AI and machine learning can help detect ransomware attacks at an early stage, allowing security teams to respond quickly and prevent the attack from spreading further. By detecting and preventing attacks early, organizations can minimize the damage caused by ransomware attacks.
Improved Accuracy
AI and machine learning algorithms are more accurate than humans in detecting ransomware attacks. They can analyze vast amounts of data in real-time and identify patterns that are not visible to the human eye. This improved accuracy can help reduce the number of false positives and ensure that security teams focus on real threats.
Cost-Effective
AI and machine learning algorithms can automate many of the tasks associated with detecting and preventing ransomware attacks, reducing the need for manual intervention. This can help organizations save time and money and improve overall efficiency.
Scalability
AI and machine learning algorithms can scale to handle large volumes of data, making them ideal for detecting and preventing ransomware attacks in large organizations with complex networks.
Continuous Learning
AI and machine learning algorithms can continuously learn and improve their ability to detect and prevent ransomware attacks. This means that over time, they become more accurate and effective at identifying and responding to ransomware attacks.
Challenges of AI and Machine Learning in Preventing Ransomware Attacks
While AI and machine learning can be effective in preventing ransomware attacks, there are also some challenges that need to be considered. These challenges include:
False Positives
AI and machine learning algorithms can generate false positives, which can lead to unnecessary
alerts and increased workload for security teams. False positives occur when the algorithms identify non-malicious activity as potentially malicious, leading to unnecessary alarms. This can be mitigated by continuously fine-tuning the algorithms to reduce the number of false positives.
Adversarial Attacks
Adversarial attacks are a type of cyberattack that specifically targets machine learning algorithms. Attackers can manipulate the data used to train the algorithms, causing them to make incorrect decisions. This can be particularly dangerous when it comes to ransomware attacks, as attackers can use this technique to evade detection and compromise the organization's systems.
Limited Understanding
Machine learning algorithms are limited by the data they are trained on. If the data used to train the algorithms is incomplete or biased, the algorithms may not be able to detect all types of ransomware attacks. Additionally, the algorithms may not be able to detect new or previously unseen types of ransomware attacks.
Cost
While AI and machine learning can be cost-effective in the long run, the initial investment can be significant. Organizations need to invest in the necessary hardware and software, as well as hire experts to develop and maintain the algorithms. This can be a significant barrier for small and medium-sized businesses.
Conclusion
Ransomware attacks are a growing threat to organizations of all sizes. These attacks can cause significant financial and reputational damage, and organizations need to take proactive steps to prevent them. AI and machine learning can be valuable tools in detecting and preventing ransomware attacks. They offer improved accuracy, scalability, and continuous learning, making them ideal for organizations with complex networks.
However, there are also challenges that need to be addressed. False positives, adversarial attacks, limited understanding, and cost are all factors that need to be considered when implementing AI and machine learning in ransomware prevention.
Despite these challenges, the benefits of AI and machine learning in ransomware prevention outweigh the drawbacks. As the threat of ransomware attacks continues to grow, organizations need to invest in technologies that can help them stay ahead of the attackers. AI and machine learning are essential tools in the fight against ransomware, and organizations that adopt them will be better positioned to prevent these attacks and protect their data and systems.