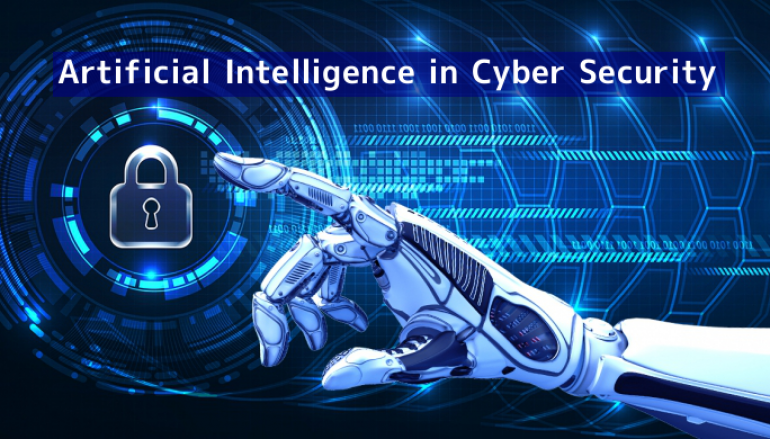
The role of artificial intelligence (AI) in cybersecurity has been growing in importance in recent years. As cyber attacks become more frequent and sophisticated, organizations are looking for new ways to protect their systems and data.
AI offers a promising solution, as it has the ability to quickly analyze large amounts of data and detect patterns that humans may miss. In this blog, we will explore the opportunities and challenges of using AI in cybersecurity.
Five Opportunities of using AI in cybersecurity
Following are a few reasons that make AI a must-have tool in cybersecurity
Enhanced Threat Detection:
AI can detect and respond to cyber threats in real-time by analyzing vast amounts of data from various sources, such as network traffic, endpoint devices, and user behavior. Machine learning algorithms can identify patterns and anomalies in this data to flag potential threats and alert security teams. This can help organizations to identify and respond to cyber threats more quickly and accurately than traditional methods.
Automation:
AI can automate routine tasks such as security alerts, incident response, and patch management, reducing the workload for security teams and freeing them up to focus on more complex security issues. By automating repetitive tasks, AI can also reduce the risk of human error, which is a common cause of security breaches.
Behavioral Analytics:
AI can analyze user behavior and detect deviations from normal patterns, which can help to identify potential insider threats or compromised accounts. For example, if an employee suddenly accesses sensitive data outside of their regular work hours, AI algorithms can detect this behavior and alert security teams.
Predictive Analytics:
AI can use historical data to identify patterns and trends that may indicate a future cyber attack, enabling organizations to take preventative measures before an attack occurs. By analyzing data such as network traffic, malware signatures, and threat intelligence feeds, AI algorithms can predict the likelihood of a cyber attack and recommend actions to prevent it.
Real-time Response:
AI algorithms can detect and respond to threats in real-time, helping to minimize the impact of an attack and reduce downtime. For example, AI can automatically quarantine infected devices or block malicious traffic to prevent a cyber attack from spreading across the network.
Five Challenges of Using AI in Cybersecurity
Major challenges that AI brings when it is used as a cybersecurity tool are;
Data Quality:
AI algorithms rely on high-quality data to operate effectively. If the data is incomplete or inaccurate, the algorithm's results may be unreliable or skewed, leading to false positives or false negatives. Therefore, organizations need to ensure that they have accurate and comprehensive data to train AI algorithms effectively.
Adversarial Attacks:
Hackers can use techniques to evade detection by AI algorithms, such as using slight variations of known attack patterns. This can make it difficult for AI to detect and respond to new and evolving threats. As a result, organizations need to continuously update and refine their AI algorithms to keep up with the evolving threat landscape.
Bias:
AI algorithms can be biased if they are trained on data that is not representative of the broader population. This can lead to inaccurate results and create security vulnerabilities. Therefore, organizations need to ensure that their AI algorithms are trained on unbiased and diverse data sets.
Explainability:
AI algorithms can be complex and difficult to understand, making it challenging for security teams to interpret the results and determine the appropriate response. Therefore, organizations need to ensure that they have the necessary skills and expertise to understand and interpret the results generated by AI algorithms.
Integration:
AI must be integrated into existing security infrastructure and processes to be effective. This can be challenging, as it may require significant changes to existing systems and processes. Therefore, organizations need to carefully plan and execute their AI integration strategy to ensure that it is seamless and effective.
Popular Case Studies
Microsoft Defender for Endpoint:
Microsoft Defender for Endpoint is a cloud-based endpoint protection platform that uses AI to detect and respond to cyber threats. The platform analyzes data from endpoints, networks, and applications to identify anomalies and suspicious behavior. Microsoft claims that the platform can detect 97% of advanced attacks, with a false positive rate of less than 0.1%.
Darktrace:
Darktrace is an AI-powered cybersecurity platform that uses machine learning to detect and respond to cyber threats. The platform analyzes network traffic, user behavior, and device activity to identify anomalies and potential threats. Darktrace claims that its platform can detect and respond to threats in real-time, without requiring human intervention.
Cylance:
Cylance is an AI-based endpoint protection platform that uses machine learning to identify and prevent cyber attacks. The platform analyzes file behavior and metadata to identify malicious code and block it before it can execute. Cylance claims that its platform can detect and prevent 99% of malware attacks.
Conclusion
The role of AI in cybersecurity is rapidly evolving, with new opportunities and challenges emerging every day. While AI offers promising solutions to the challenges of cybersecurity, it is essential to understand the limitations and potential risks of using these systems.
Organizations must carefully consider the cost, complexity, and potential biases of implementing AI systems in their cybersecurity strategies. As the threat landscape continues to evolve, AI will undoubtedly play an increasingly vital role in protecting organizations' systems and data.